Tez now renamed Google Pay is quite a successful application having clocked over 30 million transactions within 37 days of its initiation. I was though, late to the party having it installed only recently in July 2018.
Besides the cool UI and the sleek design. The application is interesting in terms of the rewards program where one can get cashback based on a certain scratch card instead of a fixed amount.
This rewards program has been of great success, being replicated across the industry. The cashback is a random event with no clear structure on what governs the cashback amount.
Data Collection
I have attached the sheet of all my transactions :
- Transactions with non-zero rewards are coloured green.
- The sole transaction in red is the one where the offer was deterministic, available on spending a specific amount on the FreshMenu application.

In an attempt to understand the exact mechanics, I made some custom columns for the data :
- Date Since Last: Days since the last transaction on Google Pay.
- Transaction Value: The amount transacted through Google Pay.
- CumTV: The Cumulative transactional value exchanged until then.
- Reward: The corresponding cashback received.
- CumR: Cumulative rewards earned till then.
- Item: Whether it is a transfer to another Google Pay user or payment to some kind of service.
- Special Offer: This column is just to identify the specific row when we got a deterministic reward.
Hypothesis #1
The reward is a function of days since the last transaction and not the value of the transaction. This makes sense if the number of active users in terms of daily or monthly is far more important than the national value.
So, if you want to know if you are up for a reward next you make a transaction is been a while. Transacting on consecutive days is likely to result in zero rewards.
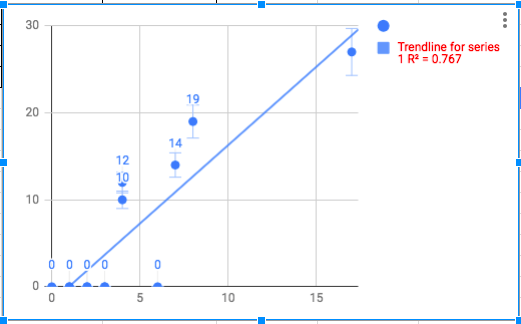
The red row was removed to maintain consistency in the type of data. Indeed, the trend is fairly apparent.
Hypothesis #2
Your cumulative reward(CumR) is a strong function of the cumulative transaction value (CumTV).
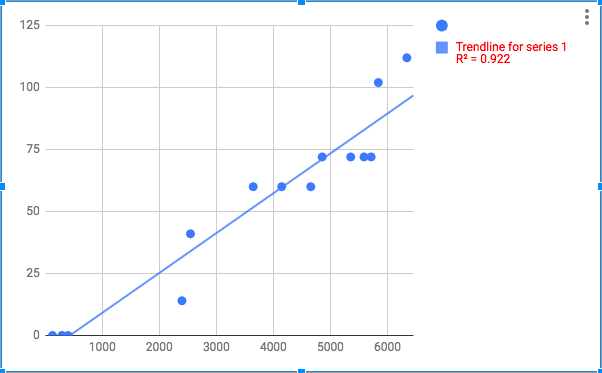
This trend is a lot stronger, it will be interesting to know if the trend slope is the same for every user or is adaptive as usage matures.
How has other’s experience been, one strong caveat has been the fact that I’m still a new user and thereby my current rewards pattern might not be the same as that of a more mature user.
Take Away
- Time as a component solves lots of issues for them.
- If you transact frequently, no need to incentivise you as they have already converted you.
- If you send it to a new person who has not transacted a lot, your likelihood of reward increases given that, you have a new connection for Google Pay and also engaging someone new into the system.
- I further strengthened the anecdotal evidence by sending some fresh transactions recently which have resulted in no rewards. Thereby, it seems like hypothesis one rather than two.
This was one of the most interesting ways for a firm to bring down the CAC or customer acquisition costs for their business. Even after ~2 years of usage, my cumulative sum of rewards is around Rs 500. Having checked around with a few of my friends, I have confirmed that their numbers at max range around 2000 bucks.
This was one of the most interesting ways to reduce the overall CAC costs by incentivising early users and tactically bringing the number down for late adopters. But these numbers are significantly lower than the CAC costs for other players like PayTm who definitely would have spent several multiple over this to not just acquire but retain customers through cashback offers.
Conclusion
This whole usage of expected value has been a great trick to engage the Indian audience. Many people don’t understand the concept of probability and that having a low probability and very high outcome is a not-so-great deal at the end of the day.
This is evident in the proliferation of gaming startups. They rely on very few winners winning large sums of money similar to lottery/gambling. People bet and gamble eyeing the large prize money. Unlikely understanding that the odds are so low. On an expected basis, irrespective of their cricketing knowledge, they are expected to lose money on each bet. It’s a losing proposition by all means.
The idea introduced by Google Pay has now been completely adopted by other players. Additionally, everyone introduces probabilistic rewards instead of fixed amounts. You are likely to increase conversions and engagement with a 10% probability of 500 bucks among 100 users over 5 for each one of them.
A very obvious concept but just works wonders when put in practicality. The key lever is that we are emotional beings at the end of the day. Moreover while logically both should be the same, the 500 possibility is sweeter in comparison to the certain 5.